Four Artificial Intelligence Threats Will Challenge the Cybersecurity Industry
Artificial intelligence (AI) and machine learning (ML) systems are becoming increasingly popular, especially with the advancements of OpenAI’s ChatGPT and other large language models (LLMs). Unfortunately, this popularity has led to these technologies being used for nefarious purposes, making AI and ML an imminent threat to security operations centers (SOCs). SOC teams need to start preparing and building threat models to stay ahead of these emerging risks.
According to a report published by Statista, the amount of big data generated is growing at a rate of 40%, and it will reach 163 trillion gigabytes by 2025. One of the primary outcomes of this explosion of data is the emergence of an artificial intelligence (AI) ecosystem. The term “AI ecosystem” refers to machines or systems with high computing power that mimic human intelligence. The current AI ecosystem features technologies like machine learning (ML), artificial neural networks (ANN), robotics, etc. In this blog post, we’ll cover five major AI threats your security operations team should plan and budget for to stay ahead of the game.
Before we get started, Artificial intelligence (AI) and machine learning (ML) are two terms often interchanged, but their differences are significant. AI is comparable to human learning, where new behaviors are adopted without reference. Conversely, ML is a subset of AI, employing predefined algorithms tailored to specific data types with expected outputs. They are fixed algorithms within AI that can learn and postulate.
Considering the fact that AI has the capability of developing new algorithms for data analysis, it is a step above ML. Consequently, it is vital to evaluate the goals and functions of an organization before determining which strategy to implement.
AI-Powered Malware is Challenging Traditional Cyber Defenses
One of the most significant threats is cybercriminals using AI to create malware. AI-powered malware can identify security gaps within an organization’s systems and quickly exploit them. This kind of attack can cause a network to shut down entirely or result in hackers surreptitiously infiltrating sensitive data.
Malware developers are increasingly using AI to evade detection by traditional antivirus tools. Cybercriminals can use deep learning techniques to create new malware variants that can evade traditional signature-based detection methods. SOC teams need to invest in advanced AI-based malware detection solutions that can identify and thwart these sophisticated attacks.
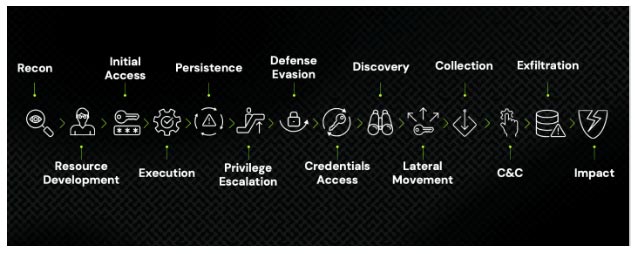
Figure 1 – A common attack chain following 14 tactics defined by the MITRE ATT&CK Matrix for Enterprise
Social Engineering Using AI is Leading to More Successful Phishing Attacks
Social Engineering: Phishing scams and social engineering attacks are commonplace; however, AI-enabled social engineering attacks can be far more potent. Cybercriminals can use AI to create voice or chatbots that mimic real people and use them to manipulate people into sharing information that can then be used to gain access to systems.
AI makes it easier for cybercriminals to launch complex social engineering attacks, in which the cybercriminal takes on the identity of someone that the target trusts, such as a senior executive or a business partner. AI manipulates natural language processing to generate content, such as emails or chat messages, that sound authentic. SOC teams should build AI models that can identify content generated by AI and distinguish between genuine messages and those that could be malicious.
If attackers can scrape data from a trove of employee LinkedIn profiles to map out the products, projects, and groups those employees work on and then feed that into an LLM, they could generate massive business email compromise (BEC) scams, sending out extremely convincing emails that look as if they are from the employees’ bosses or CFOs and that include precise details about the projects they’re working on. If an attacker managed to compromise company data and feed that into the LLM, that would make the attack look more authentic.
Data Poisoning
Data Poisoning: According to a Gartner article, data poisoning will be a considerable cybersecurity threat in the coming years. With the rise of AI, data poisoning attacks are also on the rise. The goal of these attacks is to intentionally introduce false data into an organization’s collection of data, thus skewing the results of any predictive modeling or machine learning algorithms. A form of adversarial attack, data poisoning involves manipulating training datasets by injecting poisoned or polluted data to control the behavior of the trained ML model and deliver false results.
The potential damage of backdoor attacks on machine learning models cannot be overstated. Such attacks are not only more sophisticated than straightforward injection attacks but also more perilous. By planting undetected corrupt data into an ML model’s training set, adversaries can slip in a backdoor. This hidden input allows them to manipulate the model’s actions without the knowledge of its creators. The malicious intent behind backdoor attacks can go unnoticed for extended periods, with the model functioning as intended until certain preconditions are met, triggering the attack. Hence, taking necessary measures to prevent backdoor attacks on your machine learning models is crucial.
Generating Deepfakes with AI is Leading to Massive Fraud
Generating deepfake data: Since it can create convincing imitations of human activities—like writing, speech, and images—generative AI can be used in fraudulent activities such as identity theft, financial fraud, and disinformation. AI-generated deep fakes are used to generate content that looks and appears authentic while containing false information. For instance, a deep fake video can depict someone saying or doing something they never did, leading to reputation damage, decreased credibility, and other types of harm.
Deepfake technology has been on the rise for decades, however in recent years, deep fakes have become more accessible and advanced, thanks to the development of robust and versatile generative models, such as autoencoders and generative adversarial networks; this only makes it harder for us to distinguish between what is real and what is unreal. Deepfake technology uses machine learning algorithms to analyze and learn from accurate data, such as photos, videos, and voices of people, and then generate new data that resembles the original but with some changes.
Deepfake technology uses Artificial Neural Networks (ANNs) that learn from data and carry out tasks requiring human intelligence. ANNs analyze audio, images, and videos to develop new data similar to the original yet altered. Developers use two ANNs to create deepfakes: one generates fake data, and the other discerns how convincing the data looks. The generator applies this judgment to improve its output until it deceives the discriminator, thus creating a Generative Adversarial Network (GAN).
Real-World Examples of Deepfake Technology:
- A deepfake video of former US president Barack Obama giving a speech that he never gave, created by comedian Jordan Peele.
- A deepfake video of Facebook CEO Mark Zuckerberg boasting about having billions of people’s data was created by artists Bill Posters and Daniel Howe.
- Cruise doing various stunts and jokes created by TikTok user @deeptomcruise.
- A deepfake app called Reface allows users to swap their faces with celebrities in videos and gifs.
- A deepfake app called Wombo allows users to make themselves or others sing and dance in videos.
Conclusion
AI technologies and machine learning systems are developing rapidly, and their applications in cybersecurity are continually evolving. Security operations center teams must actively prepare for the growing security threats AI poses. SOC teams must start building threat models, skill up and procure the right tools for the fight and build resilience to meet emerging challenges. In this rapidly changing technological landscape, SOC teams that are proactive in building their resilience to AI-based threats will be better positioned to protect their organizations.
The emergence of AI in security operations has added a new layer of complexity to cybersecurity. As we discussed, there are several AI-driven threats that SOC teams should be preparing for, both in terms of creating threat models and budgeting for the appropriate tools. Security layers must evolve to quarantine malicious activity and enforce preventive practices, such as least privilege access or conditional access to local resources (like cookie stores) and network resources (like web applications). Ignoring these threats could prove to be a costly mistake, potentially leading to reputational damage, data breaches, and other types of security violations. It’s time for SOC teams to get ahead of the game and proactively address these threats. The sooner you start preparing, the better positioned you will be to protect your organization from emerging AI-related risks.